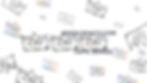
What is data science?
Data science is a multidisciplinary field that uses scientific methods, processes, algorithms, and systems to extract knowledge and insights from structured and unstructured data. It combines aspects of statistics, mathematics, computer science, and domain-specific knowledge to analyze and interpret complex data sets.
Data science involves various techniques such as data mining, machine learning, statistical analysis, and visualization to uncover hidden patterns, relationships, and trends in data. The insights gained from data science can be used to make informed decisions and predictions, identify opportunities and risks, and optimize processes and strategies across different domains such as business, healthcare, finance, and more.
The history of data collection dates back to ancient times. For example, the ancient Egyptians kept detailed agricultural production records, while the ancient Greeks collected data on population size and mortality rates.
However, the modern era of data collection began in the late 19th and early 20th centuries with the development of statistical methods and the growth of government and industry. The first national census was conducted in the United States in 1790. Still, it was not until the late 1800s that the census collected more detailed data on population characteristics such as ethnicity and occupation.
In the early 20th century, the development of statistical methods such as sampling and hypothesis testing allowed for more rigorous and systematic data collection. This led to the growth of government agencies such as the Bureau of Labor Statistics and the National Institutes of Health, which collected data on such issues as employment and health.
With the advent of computers in the mid-20th century, data collection became more efficient and sophisticated. Large-scale surveys and experiments could be conducted more quickly and accurately, and data could be processed and analyzed more efficiently.
Today, data collection continues to evolve with technological advances such as machine learning and artificial intelligence. Data cleaning and preparation is the process of identifying and correcting errors, inconsistencies, and incomplete or irrelevant data in a data set. It is a critical step in data analysis and is necessary to ensure that the data is accurate, consistent, and reliable.
The process of data cleaning and preparation typically involves the following steps:
1. Data collection: Collecting data from various sources such as surveys, databases, and social media platforms.
2. Data integration: Combining data from different sources into a single data set.
3. Data inspection: Examining the data for errors, inconsistencies, and missing values.
4. Data cleaning: Correcting errors, resolving inconsistencies, and filling in missing values.
5. Data transformation: Converting data into a suitable format for analysis.
6. Data reduction: Reducing the size of the data set by removing irrelevant or redundant data. 7. Data sampling: Selecting a representative subset of the data for analysis.
Data cleaning and preparation is time-consuming and requires careful attention to detail. However, ensuring that the data is accurate and reliable and that the insights gained from data analysis are meaningful and actionable is essential.
Companies, businesses, and governments collect data in various ways, including:
1. Surveys: Companies and governments often conduct surveys to gather individual data. These surveys can be conducted online, over the phone, or in person.
2. Online tracking: Companies track users' online activities and collect data through cookies, beacons, and other tracking technologies. This data can include browsing history, search queries, and demographic information.
3. Social media: Companies can collect data from social media platforms like Facebook, Twitter, and LinkedIn. This data can include user profiles, posts, and interactions.
4. Public records: Governments collect data from public documents such as birth and death certificates, property records, and court proceedings.
5. Sensors: Companies and governments use sensors to collect data from the physical world, such as traffic patterns, weather conditions, and energy usage.
6. Transactional data: Companies collect data on customer transactions, such as purchases, returns, and exchanges.
7. Data brokers: Companies purchase data from data brokers who collect data from various sources and sell it to businesses and governments.
It's important to note that data collection practices should follow ethical and legal guidelines, such as obtaining informed consent from individuals and protecting personal information.
Yes, the information collected through various means can be used to manipulate people. Companies and governments can use this information to influence people's opinions, decisions, and behaviors. For example, companies can use targeted advertising based on users' browsing and search history to influence their purchasing decisions. Governments can use surveillance and monitoring data to control public opinion or suppress dissent.
It's important to note that there are ethical and legal guidelines regarding the use of data. Data misuse can lead to privacy violations, discrimination, and other harmful consequences. Data collection is sometimes regulated by laws such as the General Data Protection Regulation (GDPR) in the European Union and the California Consumer Privacy Act (CCPA) in the United States.
Data collection is crucial for developing algorithms and artificial intelligence (AI). AI systems are trained on large data sets to learn patterns and make predictions based on that data. The more available data, the more accurate and reliable the predictions and decisions made by AI systems.
Data collection also helps improve the performance of algorithms. By collecting data from various sources, algorithms can be trained on diverse data sets that reflect a range of circumstances and scenarios. This helps algorithms perform better in real-world situations and adapt to new challenges.
In addition, data collection helps improve the accuracy of machine learning models. As more data is collected and added to a model, the model becomes more refined and better able to make accurate predictions.
Overall, data collection is essential for developing and improving algorithms and AI systems. With data, algorithms and AI systems would have the information they need to learn, improve, and make accurate predictions.
Here are some ways to protect yourself from the unwarranted or unwanted data collection:
1. Use privacy settings: Adjust the privacy settings on your devices and accounts to limit the amount of data that can be collected. For example, you can turn off location tracking, limit ad tracking, and control what personal information is shared.
2. Use a VPN: A virtual private network (VPN) can help protect your online privacy by encrypting your internet connection and masking your IP address.
3. Use anti-tracking tools: Several browser extensions and anti-tracking tools can help block tracking cookies and other tracking technologies.
4. Be cautious online: Be mindful of what you share online and avoid giving out personal information unnecessarily. Be wary of phishing scams and other attempts to trick you into giving out personal information.
5. Read privacy policies: Read the privacy policies of websites and apps before using them to understand how your data will be used and shared.
6. Use strong passwords: Use strong, unique passwords for each of your accounts to prevent unauthorized access.
7. Review your data: Regularly check the data companies and apps have collected about you and delete any unnecessary or unwanted data.
By taking these steps, you can help protect your personal information and reduce the amount of data that is collected about you without your knowledge or consent.